Learning About Agents Using Graph Transformers
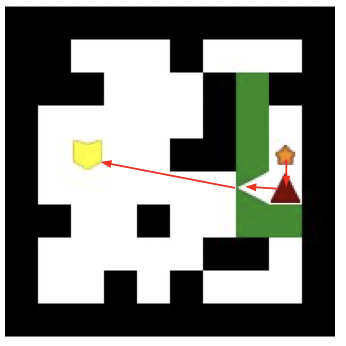
Description: Humans have a rich capacity to infer mental states of others by observing their actions. In fact, cognitive scientists have found that even very young infants expect other agents to have object-based goals, to have goals that reflect preferences, to engage in instrumental actions that bring about goals, and to act efficiently towards goals. These are all capabilities that are part of what we call Theory of Mind.
On the other hand, state-of-the-art AI systems still struggle to capture the ‘common sense’ knowledge that guides prediction, inference and action in everyday human scenarios. The Baby Intentions Benchmark (BIB) is a comprehensive benchmark that captures the generalisability of human reasoning about other agents. It adapts experiments from studies with infants and therefore it adopts their same evaluation paradigm, the Violation of Expectation. Designing a model able to perform well in these benchmark tasks represents a big step towards AI systems that reason like humans.
Goal: Modify the CNN encoder in the baseline architecture with Graph Neural Networks (GNN) and/or Graph Transformers. Explore different GNN layers and Graph Transformer architectures.
Supervisor: Matteo Bortoletto
Distribution: 70% implementation, 30% analysis
Requirements: Good knowledge of deep learning, strong programming skills in Python and PyTorch, keen interest in GNNs and Transformers.
Preferable: knowledge of GNN libraries such as DGL or PyTorch Geometric.
Literature:
Gandhi, Kanishk, et al. 2021. Baby Intuitions Benchmark (BIB): Discerning the goals, preferences, and actions of others. Advances in Neural Information Processing Systems (NeurIPS) 34, p.9963-9976.
Jiang, Zhengyao, et al. 2021. Grid-to-Graph: Flexible Spatial Relational Inductive Biases for Reinforcement Learning. arXiv:2102.04220.
Min, Erxue, et al. 2022. Transformer for Graphs: An Overview from Architecture Perspective. arXiv:2202.08455.
Rabinowitz, Neil, et al. 2018. Machine theory of mind. International conference on machine learning. PMLR.
Wu, Zhanghao, et al. 2021. Representing long-range context for graph neural networks with global attention. Advances in Neural Information Processing Systems (NeurIPS) 34, p.13266-13279.
Wu, Zonghan, et al. 2020. A comprehensive survey on graph neural networks. IEEE transactions on neural networks and learning systems 32(1), p.4-24.