Revisiting Data Normalization for Appearance-Based Gaze Estimation
Xucong Zhang, Yusuke Sugano, Andreas Bulling
Proc. ACM International Symposium on Eye Tracking Research and Applications (ETRA), pp. 1–9, 2018.
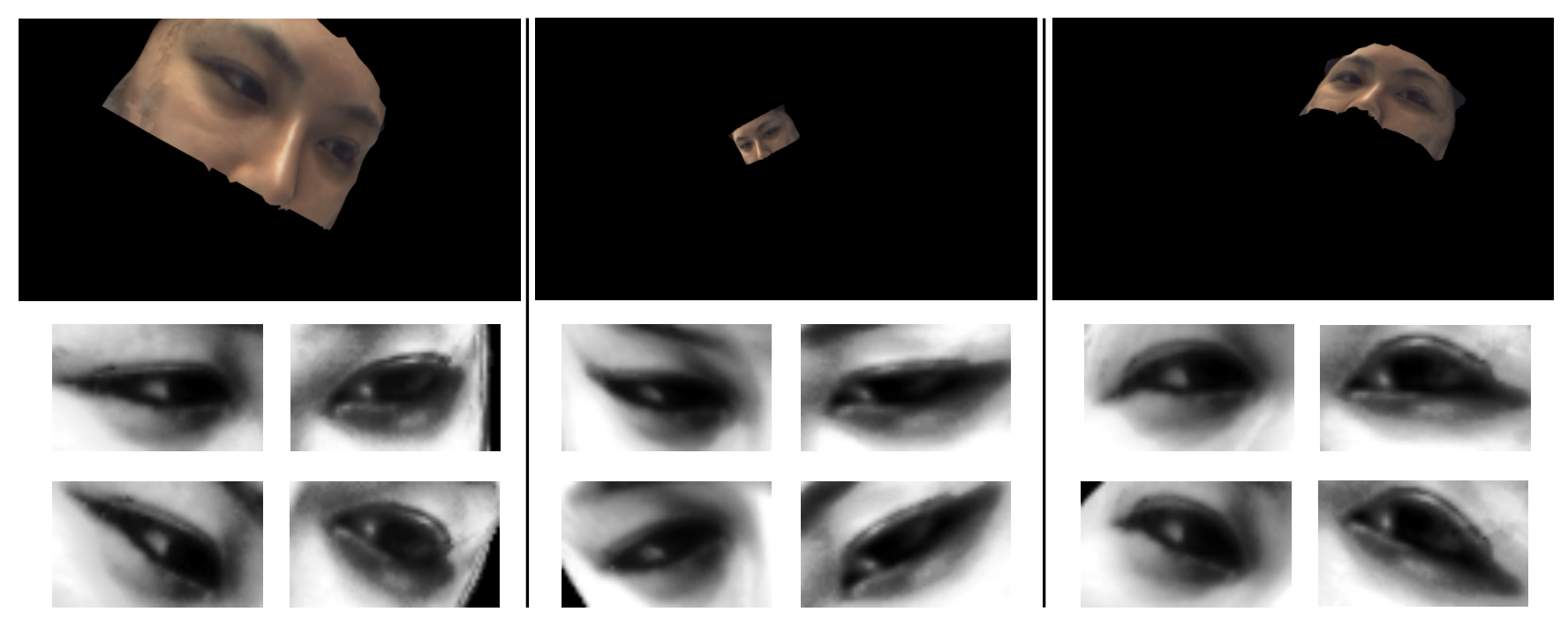
Abstract
Appearance-based gaze estimation is promising for unconstrained real-world settings, but the significant variability in head pose and user-camera distance poses significant challenges for training generic gaze estimators. Data normalization was proposed to cancel out this geometric variability by mapping input images and gaze labels to a normalized space. Although used successfully in prior works, the role and importance of data normalization remains unclear. To fill this gap, we study data normalization for the first time using principled evaluations on both simulated and real data. We propose a modification to the current data normalization formulation by removing the scaling factor and show that our new formulation performs significantly better (between 9.5% and 32.7%) in the different evaluation settings. Using images synthesized from a 3D face model, we demonstrate the benefit of data normalization for the efficiency of the model training. Experiments on real-world images confirm the advantages of data normalization in terms of gaze estimation performance.Links
Paper: zhang18_etra.pdf
BibTeX
@inproceedings{zhang18_etra,
author = {Zhang, Xucong and Sugano, Yusuke and Bulling, Andreas},
title = {Revisiting Data Normalization for Appearance-Based Gaze Estimation},
booktitle = {Proc. ACM International Symposium on Eye Tracking Research and Applications (ETRA)},
year = {2018},
pages = {1--9},
doi = {10.1145/3204493.3204548}
}