Neural Photofit: Gaze-based Mental Image Reconstruction
Florian Strohm, Ekta Sood, Sven Mayer, Philipp Müller, Mihai Bâce, Andreas Bulling
Proc. IEEE International Conference on Computer Vision (ICCV), pp. 245-254, 2021.
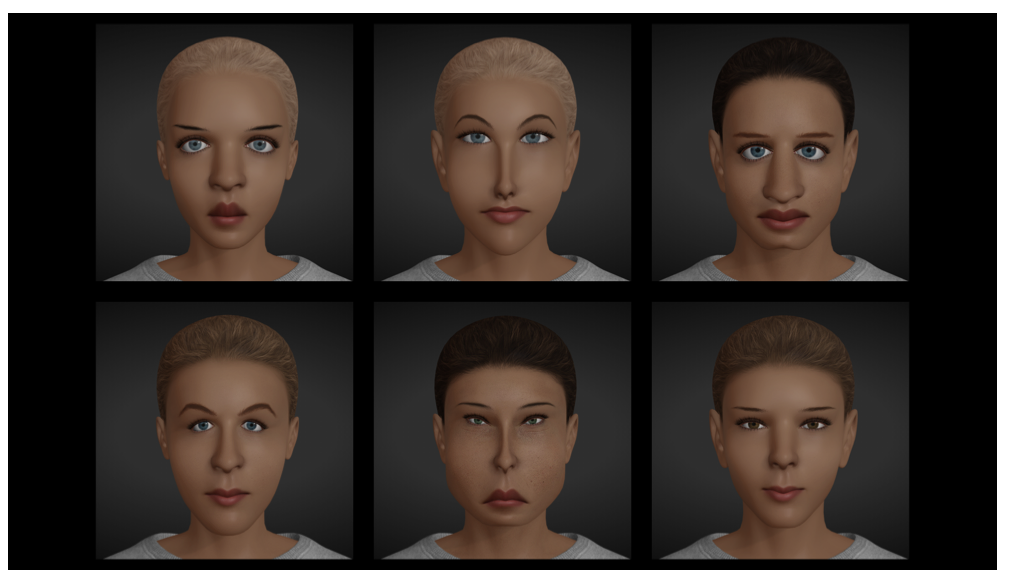
Abstract
We propose a novel method that leverages human fixations to visually decode the image a person has in mind into a photofit (facial composite). Our method combines three neural networks: An encoder, a scoring network, and a decoder. The encoder extracts image features and predicts a neural activation map for each face looked at by a human observer. A neural scoring network compares the human and neural attention and predicts a relevance score for each extracted image feature. Finally, image features are aggregated into a single feature vector as a linear combination of all features weighted by relevance which a decoder decodes into the final photofit. We train the neural scoring network on a novel dataset containing gaze data of 19 participants looking at collages of synthetic faces. We show that our method significantly outperforms a mean baseline predictor and report on a human study that shows that we can decode photofits that are visually plausible and close to the observer’s mental image. Code and dataset available upon request.Links
doi: 10.1109/ICCV48922.2021.00031
Paper: strohm21_iccv.pdf
Code: Available upon request.
Dataset: Available upon request.